Creating a comprehensive weed image dataset for tomato fields is essential for training deep learning algorithms, such as Convolutional Neural Networks (CNNs). These CNN models are particularly effective when trained on RGB images of various weed species. In our study, we focused on both monocotyledonous (Cyperus rotundus L., Echinochloa crus galli L., Setaria verticillata L.) and dicotyledonous (Portulaca oleracea L., Solanum nigrum L.) weeds, capturing the diversity and complexity needed for precise weed identification.
The process began with extensive fieldwork in the commercial tomato fields of Badajoz, where we captured a large number of images under varying conditions. This area, known for its intensive tomato cultivation, provided an ideal setting to gather a diverse dataset. We took images of weeds at different growth stages, from seedlings to mature plants, and under various lighting conditions to ensure that our dataset was comprehensive.
Using high-resolution cameras, we meticulously photographed the weeds from multiple angles to capture the unique characteristics of each species. The collected images were then annotated with details such as weed species, growth stage, and geolocation data. This careful documentation was crucial for creating a high-quality dataset that would be suitable for training our CNN models.
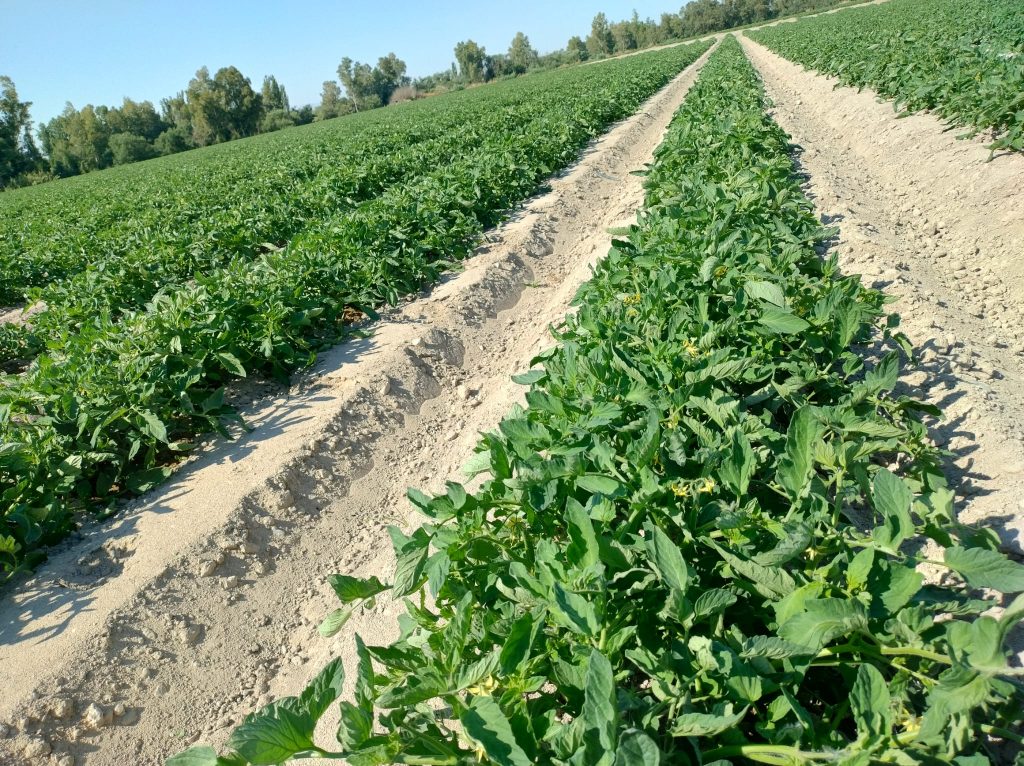
The annotated images were preprocessed to ensure uniformity in size and format, making them ready for the training phase. Our CNN models were then trained on these RGB images, learning to distinguish between the monocotyledonous and dicotyledonous weeds. The models were rigorously validated and tested to ensure high accuracy and robustness in weed identification.
Through this meticulous process, our study highlights the importance of a well-constructed image dataset in enhancing the precision, efficiency, and sustainability of agricultural practices. By focusing on both monocotyledonous and dicotyledonous weeds and leveraging advanced deep learning techniques, we aim to significantly improve site-specific weed management in tomato cultivation. This approach not only supports higher crop yields but also promotes sustainable farming by reducing reliance on chemical herbicides.
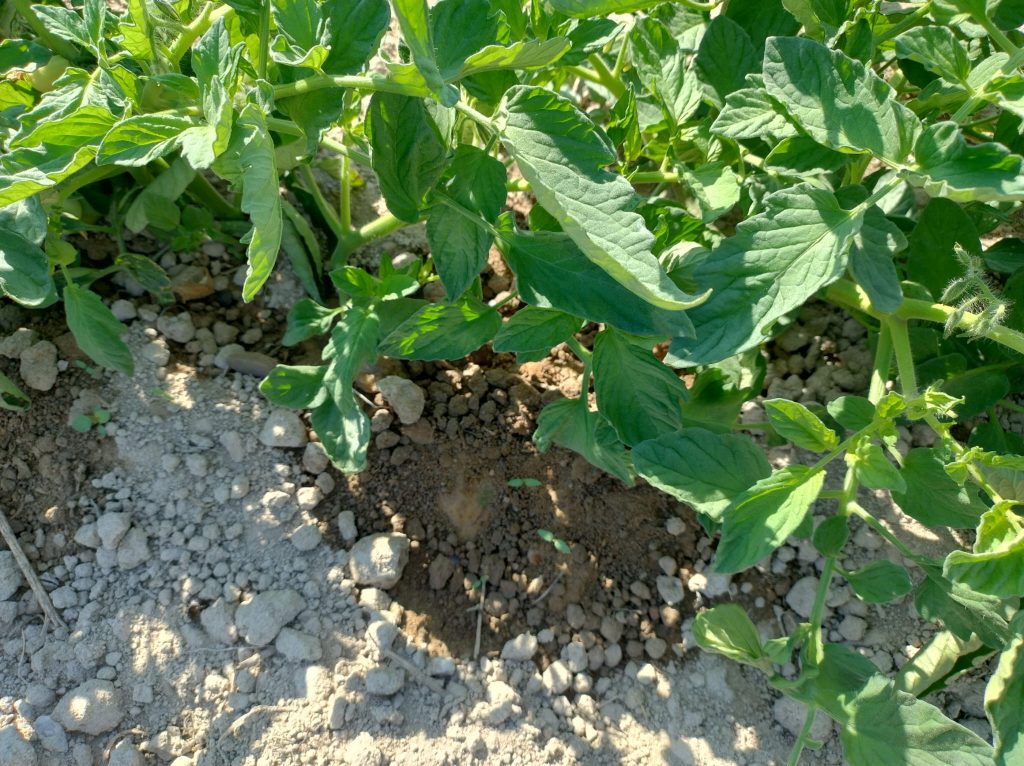
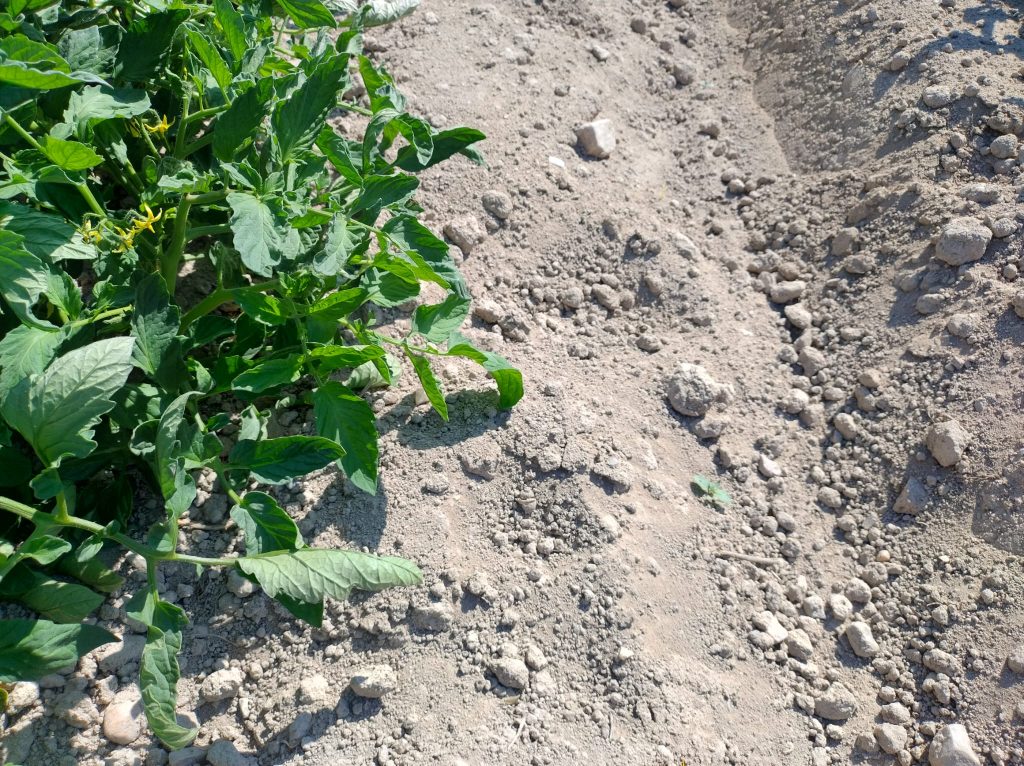
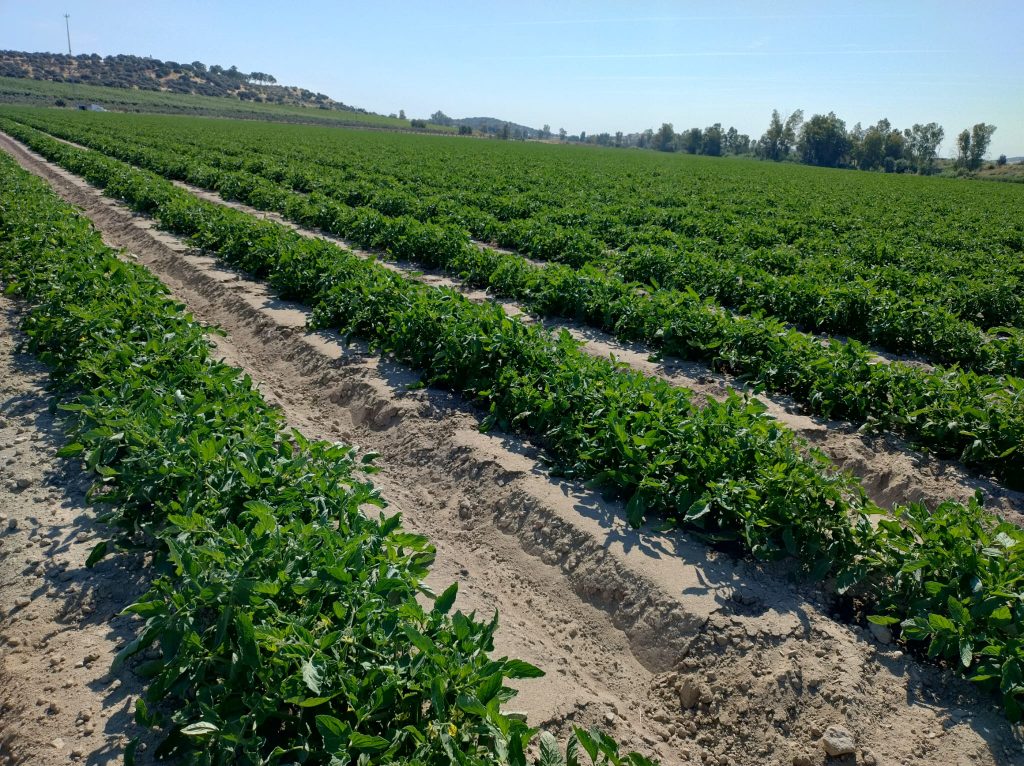