Take a closer look at these images (at the end of the text) from the experimental tomato commercial fields in Badajoz, where a significant weed infestation is evident. This infestation is a result of the reduced efficacy of traditional herbicide treatments, underscoring the urgent need for more effective weed management strategies. In response to this challenge, the SWEET project by CSIC is using artificial intelligence for sustainable weed management. The team at CAR-CSIC is applying advanced deep-learning techniques to these fields, enabling precise weed identification and control. By utilizing high-resolution imagery and machine learning algorithms, the system can distinguish between tomato plants and various weed species with remarkable accuracy. This allows for targeted herbicide application, significantly reducing chemical use and promoting eco-friendly farming practices.
Despite their effectiveness, herbicides’ overuse has led to the development of resistant weed species, reducing the overall efficacy of herbicide treatments. This growing resistance makes traditional blanket herbicide application less reliable and increases the need for alternative strategies. Site-specific weed management (SSWM) emerges as a vital approach in this context, focusing on treating only the affected areas rather than the entire field. This method not only conserves herbicides but also mitigates the risk of developing further resistance.
Deep learning, a subset of machine learning, involves training artificial neural networks with numerous layers to recognize patterns and make decisions. In the context of these tomato fields, drones and ground-based sensors capture high-resolution images, which are then processed by these neural networks. The AI system analyzes the images to detect and classify different types of weeds among the tomato plants. One of the key techniques used in this process is convolutional neural networks (CNNs), which are particularly effective for image recognition tasks. CNNs can automatically and accurately identify the features that differentiate weeds from crops. This precise identification is crucial for site-specific weed management, where only the areas with weed infestations are treated, reducing the overall use of herbicides. Additionally, the project employs reinforcement learning, where the AI system learns to make decisions based on trial and error. This method enhances the efficiency of weed control strategies over time, as the system continuously improves its accuracy and effectiveness.
The implications of these technologies extend beyond weed control. By reducing the use of herbicides, the project promotes sustainable farming practices, preserving soil health and reducing environmental impact. Moreover, the data collected can be used to optimize other aspects of crop management, such as irrigation and nutrient application, further enhancing productivity and sustainability. It is crucial to bear in mind that weeds are a major challenge in agriculture, competing with crops for vital resources such as nutrients, water, and light. Uncontrolled weeds can reduce crop yields by up to 60%, making effective weed management essential for productivity. The AI-driven approaches being tested here aim to revolutionize weed control, improving efficiency and sustainability.
In conclusion, the reduced efficacy of traditional herbicide treatments due to resistant weed species highlights the importance of adopting site-specific weed management strategies. By leveraging advanced AI technologies, the SWEET project is pioneering a more precise and sustainable approach to weed control, ensuring the long-term health and productivity of agricultural systems.

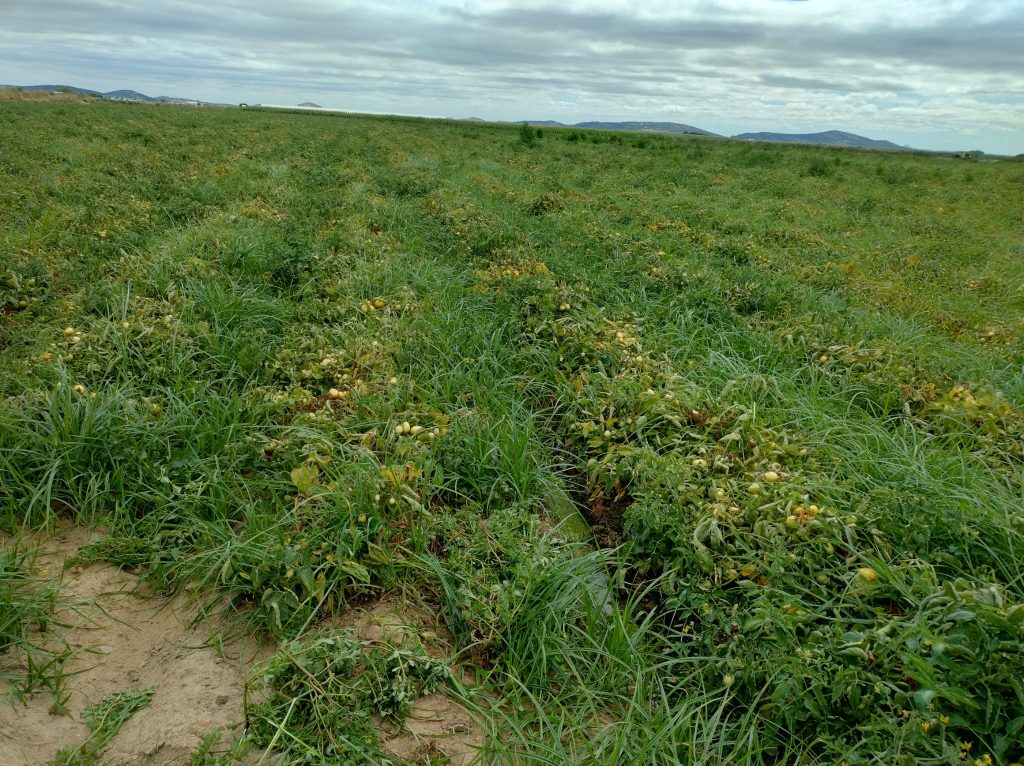
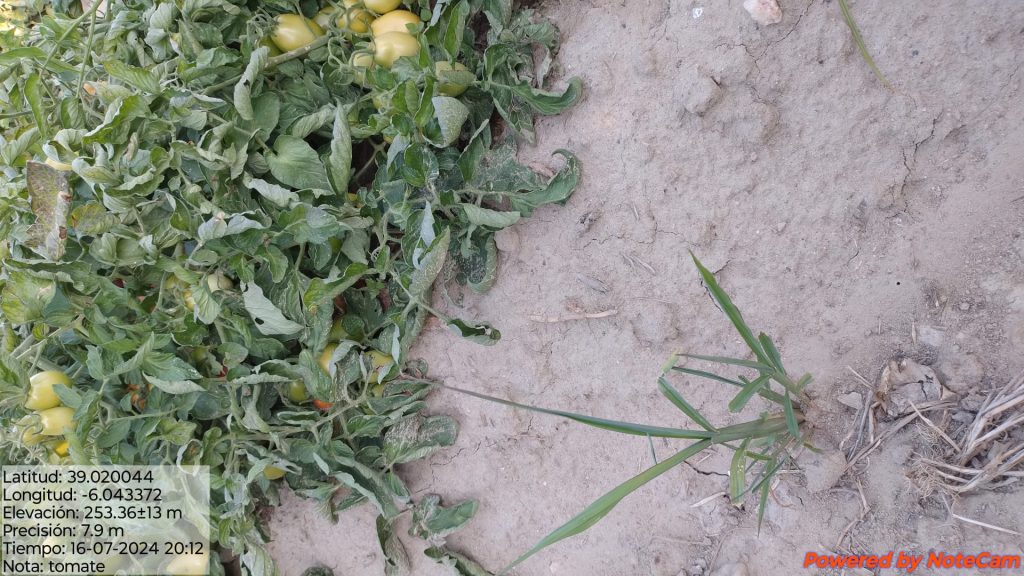
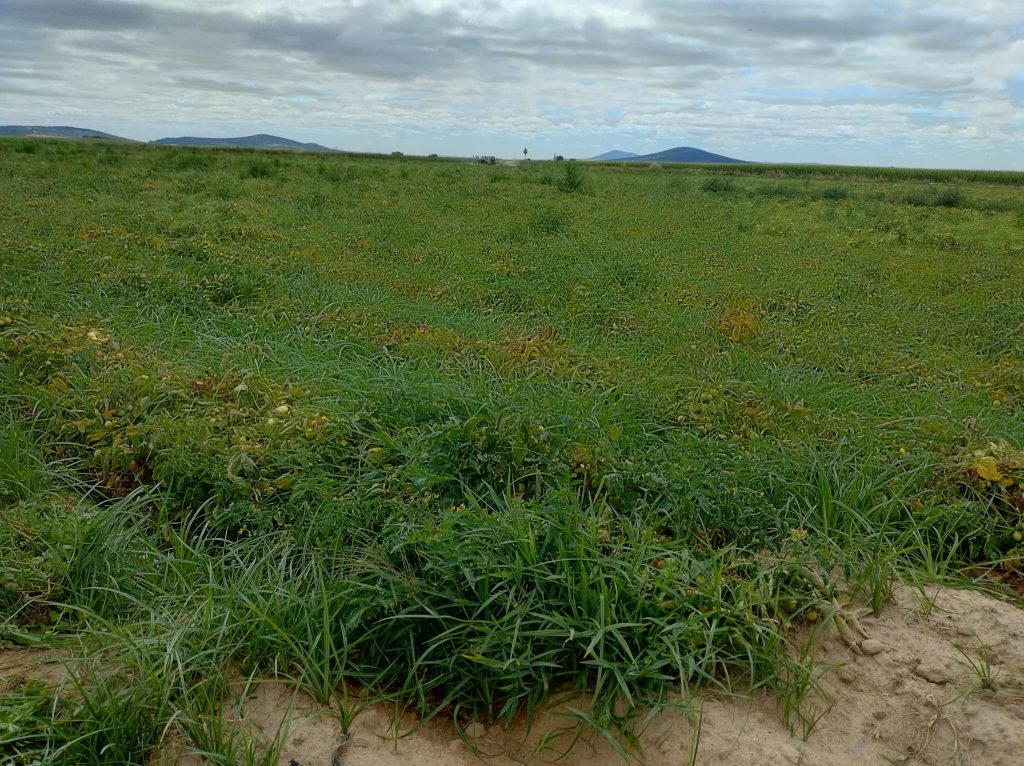